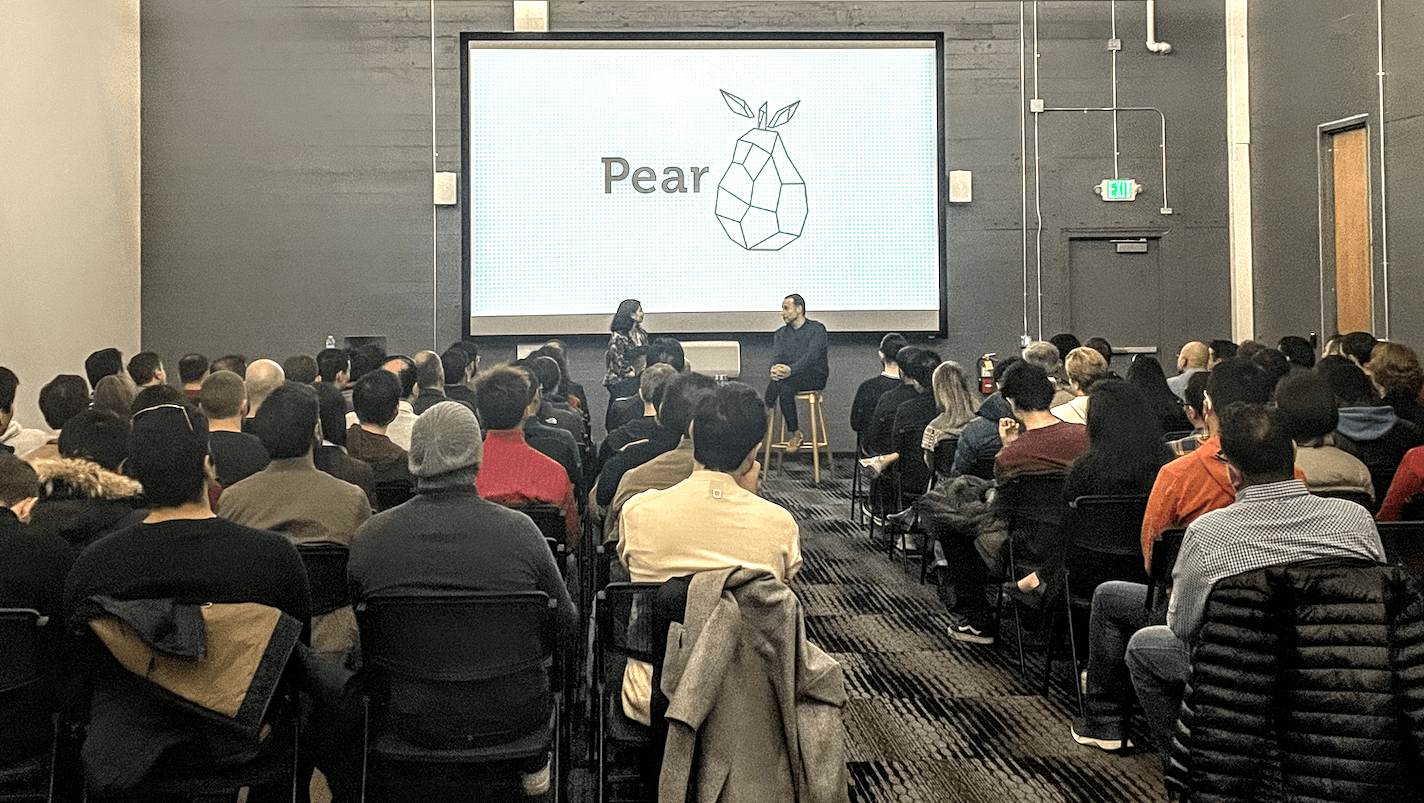
On March 1st, Pear’s Aparna Sinha hosted a fireside chat with Hussein Mehanna, SVP of Engineering for AI/ML at Cruise for a discussion on next generation AI/ML technologies. Hussein has a long and deep history of innovation in machine learning engineering spanning speech recognition, language models, search, ads, and ML platforms at companies including Google, Facebook and Microsoft. He is currently focused on ML-driven robotics, especially autonomous vehicles at Cruise.
This is the first in a series of AI/ML events Pear is hosting. To hear about future events, please sign up for our newsletter and keep an eye on our events page.
The exciting conversation lasted for over an hour, but below is a summary with some highlights from the talk between Aparna and Hussein:
Q: You’ve been building products at the forefront of AI throughout your career, from search, to speech to ML platforms and now robotics and autonomous vehicles. Tell us a little bit about your journey, and the evolution of your work through these products?
A: My journey began with a scholarship for neural networks research in 2003, followed by a role at Microsoft. I eventually joined Facebook and worked on Ads that pushed the limits of ML and from there moved to a more broadened role of working with ML platforms across the company. I then joined Google Cloud’s AI team to explore disruption of enterprise through ML. I learned over the years that robotics is the biggest field facing disruption with machine learning, and autonomous vehicles is the biggest application of that. So I joined Cruise both out of interest in robotics and a pure interest in cars.
Q. Ads, in fact, also at Google was the birthplace of a lot of the advanced AI. And now AI is absolutely into everything.
A: Absolutely. There was a system in Google Ads called Smart ass. It was actually one of the first known large scale machine learning systems. And the person who developed them, Andrew Moore, eventually became my manager at Google Cloud AI. You’d be surprised how many lessons to be learned from building machine learning for ads that you could implement in something as advanced as autonomous vehicles.
You’d be surprised how many lessons to be learned from building machine learning for ads that you could implement in something as advanced as autonomous vehicles.
Q: We are seeing the emergence of many AI-assistive products, co-pilot for x, or auto-pilot for y. But you’ve spoken about AI-native products. Are AI-assistive products and AI-native products fundamentally different?
A: Yes, they are. An AI-native product is one that cannot exist, even in MVP form, without machine learning. Examples include autonomous vehicles or speech recognition software like Alexa. On the other hand, AI-assistive products can help humans in various ways without necessarily using machine learning. In fact, Google search, people may not know that, but Google Search started with more of a data mining approach than machine learning.
Q: What is the gap between building an AI-assistive product versus an AI-native product?
A: The gap is huge. Building an AI-native product assumes full autonomy, while building an AI-enhanced product assumes a human being will still be involved. For example, the technology used for driver-assist features (level 1-3 autonomy) versus fully autonomous driving (level 4-5 autonomy) require vastly different approaches and parameters. Autopilot is actually classified as Driver Assist. But then once you remove the driver completely, from behind the wheel, you get into level 4, level 5, autonomy. Level 5 is maybe less dependent on a predefined map, you could just throw the robot anywhere, and they’ll figure its way. It’s very important for founders, entrepreneurs, product managers to understand, are they building something that assists human beings, and therefore assumes a human being, or something that completely replaces them.
Q: Where do generative AI and GPT technologies fall on the spectrum?
A: Generative AI and GPT – so far – are human-assisted technologies that require a human being to function properly. Today, they are not designed to replace humans like technologies used for level 4-5 autonomy.
Q: At a high level, what are the components and characteristics of a fully autonomous system? I’ve heard you call it an AI brain.
A: So let me project the problem first, from a very high level on driving, because I suspect most of us have driven before. For a full autonomous system the first component is perception, you need to understand the environment, and essentially describe the environment as the here and now. This is a vehicle, it’s heading this direction, with this velocity; here’s a pedestrian, he or she is x distance away from you, and they’re heading that way, and that’s their velocity. Here’s a pile of dirt. And here’s a flying plastic bag. And here’s something that we don’t know what it is, right? So perception is extremely important. Because if you don’t understand the environment around you, you don’t know how to navigate it.
Now, what’s very, very important about perception is that you can’t build a perception system that is 100% perfect, especially a rich system that describes all sorts of things around you. And so one of the lessons we’ve learned is, you can build multiple levels of perception. You can build a level of perception that is less fine grained. A machine learning system that just understands these two categories can generalize better. And it’s very important for your perception system to have some self awareness so that it tells you the rich system is confused about this thing here. So let’s go to the less sophisticated system and understand whether it’s something that is safe to go through or go around. Now the reason why you need the rich system is because it gives you rich information. So you can zip through the environment faster, you can finish your task faster. And if your rich system is accurate, let’s say x percent of the time with a little bit of unsureness, then it’s okay to drive a little bit slower using the less rich, less refined system. So that’s number one about perception.
The second component of autonomous driving is prediction, which involves understanding how agents in the environment will interact with each other. For example, predicting whether a car will cut you off or slow down based on its behavior. However, predicting the future behavior of other agents is dependent on how your car will behave, leading to an interactive loop. We’ve all been in this situation, you’re trying to cross the road, there seems to be a car coming up. If you’re assertive, very likely, in crossing the road, the car will stop. Or if they’re more assertive, you’ll probably back off. At Cruise, we no longer separate the prediction system from the maneuver planning system. We have combined them to decide jointly on what is the future behavior of other agents and our future, to solve extremely complicated interactive scenarios, including intersections with what they call a “chicken dance” where cars inch up against each other. We now call this the “behaviors” component.
The third component is motion planning and controls, where the car starts actually executing on its planned trajectory with smoothness. This component plays a huge role in delivering a comfortable ride because it can accurately calculate the optimal braking speed that reduces jerk (or discomfort). Most of our riders feel the difference immediately compared to human driving where a human driver could pump the breakers harder than necessary. Simulation is also a critical component of autonomous driving, which is often considered only as a testing tool but is, in fact, a reverse autonomous vehicle problem. Simulation involves building other agents that behave intelligently, such as human drivers, pedestrians, and cyclists. At Cruise, we have seen massive improvements in simulation since we have taken a big chunk of our AI and Autonomous Vehicle talent and put them in simulation. The technology we are working on is generalizable and broadly applicable to any robotics problem, such as drones and robots inside warehouses.
I like to tell people that by joining Cruise, people are building their ML-driven robotics career, which can be applied to many other places. The stack of perception, prediction, maneuvering, and simulation can be scaled to other robotics problems. Robotics is pushing AI technology to its limits as it requires reasoning, self-awareness, and better generative AI technologies.
Robotics is pushing AI technology to its limits as it requires reasoning, self-awareness, and better generative AI technologies.
Q: The concepts you described here of predicting and simulating, giving your AI system a reasoning model, and self awareness, in terms of how confident it should be. These are lacking in today’s generative AI technologies. Is this a future direction that could produce better results?
A: I do believe robotics is going to push AI technology to its limits, because it is not acceptable that you build a robot that will do the operation 99% of the time, correct, the 1% of the time can introduce massive friction.
Generative AI is very impressive, because it sort of samples a distribution of outputs, for a task that is not extremely well defined. There’s so many degrees of freedom, it’s like, give me a painting about something. And then it produces a weird looking painting, which in reality is an error. But you’re like, Wow, this is so creative. That’s why I say generative AI and particularly chatGPT do not replace human beings, they actually require a human operator to refine it. Now it may reduce the number of human beings needed to do a task. But it’s L3 at best.
Now, in order to build an L4 and above technology, especially if it has a sort of a massive safety component. Number one, you need various components of this technology to have some self awareness of how sure they are. And us as humans, we actually operate that way with a self awareness of uncertainty. L4 technologies are not going to be able to be certain about everything. So they have to be self aware about the uncertainty of whatever situation they’re in. And then they have to develop sort of policies to handle this uncertainty versus chance it up to tell you whatever, statistically, it’s learned without self awareness of its accuracy.
Q: What do you think about the combination of generative AI and a human operator in various fields such as education and healthcare?
A: Using generative AI alongside a human operator can result in an incredible system. However, it’s important to be mindful of the system’s limitations and determine whether you’re creating an L3 system with more degrees of freedom or an L4 system with no human oversight. In the field of education, generative AI can be a valuable tool, but it’s crucial to acknowledge that education is a highly sensitive area. On the other hand, in healthcare, as long as a physician reviews the outcomes, there are considerable degrees of freedom.
Q: I’ve heard great reviews from riders using Cruise’s service in San Francisco. What was your experience like in a driverless ride?
A: My first driverless ride was in a Chevy Bolt vehicle with a decent sensor package on top. At first, I felt a little anxious, but quickly realized that the vehicle was an extremely cautious driver that obeyed stop signs and braked very well. The vehicle optimized the braking and turning speeds, which made me feel safe and comfortable. I have seen the same reaction from family and friends who have ridden in the vehicles.
I think that the new Origin car is amazing and looks like the future. It’s a purposely built car for autonomy with no steering wheel and has two rows of seating facing each other. I believe that it’s going to be a very different experience from the current driverless rides, as it becomes real that there’s no driving and the car is really moving itself. The feedback from multiple people who have experienced it is that it’s as big as their first driverless ride. I also think that people will love the Origin car because it’s more comfortable and cautious than any vehicle with a driver, and it looks like the future. The first version of the Origin car should be deployed this year, and I hope that many people will have the opportunity to experience it and enjoy it within the next year or two.
Q: What are some open questions and unsolved problems as we move forward in building autonomous vehicles?
A: One open question is how to move towards end-to-end learning for autonomous vehicles, which would involve creating a single, large machine learning model that takes in sensor inputs and produces control signals, rather than the current system, which is heavily componentized. Another question is how to create an equivalent to the convolutional operator, a key component in computer vision, for autonomous vehicles. This is still an early stage field that requires significant investment to develop.
Q: At Facebook, you pioneered a new approach to AI platforms that then also later permeated our work at Google Cloud. And I think it was a very meaningful contribution. Can you explain why platforms are important for machine learning productivity?
A: I pioneered a new approach to AI platforms at Facebook that focused on productivity and delivering machine learning models quickly. I believe that productivity is key for successful machine learning because it allows for quick iteration and a faster feedback loop. In my opinion, platforms are the best mechanism to deliver machine learning models quickly and make machine learning a reality. I believe what is much more powerful than building one model that is centralized, that serves everybody is to empower everybody to build the models they want, and to tweak them, and to tune them the way they like. And that’s where a machine learning platform comes in. And I do believe that is very much true in our organization. And I’ve seen that happen at Facebook, where at one point, around 2017, we had 20% of the company, either interacting or building machine learning models one way or another.
Q: In summary, are we at an inflection point in machine learning? Can autonomous systems approaches influence responsible AI more broadly?
A: I believe that we are at an inflection point where machine learning is expected to have a massive impact on multiple fields, including autonomous vehicles, robotics, and generative AI. Robotics is pioneering this concept of reasoning and understanding the environment and incorporating it, simulating it, and building your machine learning system to be accurate enough and understand the externalities. All of it is on this foundational bedrock of having great platforms which will enable quick iteration and a faster feedback loop.
I also believe that the advanced work happening in robotics and autonomous vehicles will influence the future of AI, potentially leading to a more holistic and safe system that is oriented towards reasoning. In my opinion, one potential impact of autonomous vehicle technology on machine learning is around responsible AI. We should have one strategy for product safety, rather than separate strategies for product safety and ML safety. As an autonomous vehicle engineer, I spend more time evaluating the effectiveness of the system than building and tuning the ML model. The ability to evaluate the system effectively will become increasingly important, and I hope that there will be a generation of ML engineers that are used to doing so.
I believe that we are at an inflection point where machine learning is expected to have a massive impact on multiple fields, including autonomous vehicles, robotics, and generative AI.
We’d like to extend our sincerest thanks to Hussein Mehanna for joining us for this insightful chat. His expertise and experience in the field provided valuable insights into the current and future states of AI/ML. We look forward to hosting more conversations on AI, so please keep an eye on our events page!