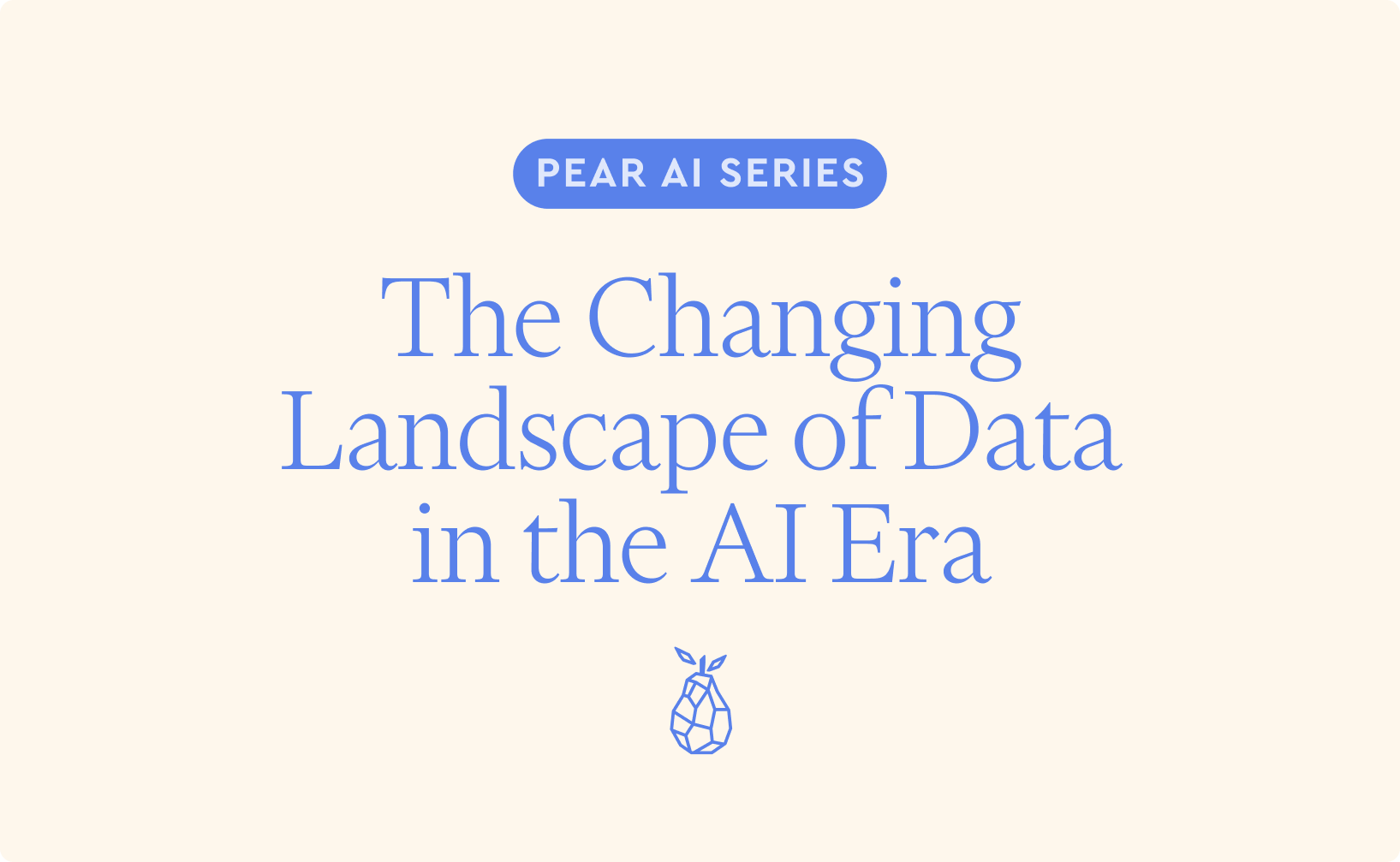
Data has always been the lifeblood of business. In the era of artificial intelligence, it is all the more important. The quality and quantity of data directly determines the performance of AI models and the insights they generate. As AI becomes more integrated across industries, data pipelines and architectures are rapidly evolving to meet these new challenges. In this article, we’ll delve into how data companies are adapting and the opportunities that lie ahead.
The Primacy of Data in AI
In the past, there’s been a great focus on the quality and complexity of the application itself. Today, the spotlight has shifted to the AI model and its underlying data. With techniques like fine-tuning, the same augmented dataset can be used to power multiple applications. This change has highlighted the vital importance of data in an AI-driven landscape.
The stakes are also higher now. In domains like healthcare and legal, where AI is being applied to critical decisions, high-quality data is non-negotiable. The human experts who provide feedback and label data need to be highly skilled. This underscores a shift in focus from the quantity, to the quality of data.
Why Data Quality Matters
So why exactly is high-quality data so crucial for AI?
Fine-tuning models entirely depends on the data used. Higher quality data also enables the use of smaller, more efficient models when 1) there are fewer errors in the data, 2) fewer features are needed to explain underlying patterns, and 3) overfitting to noise is less likely. This leads to improved performance, faster training times, and significant savings in compute costs.
As a result, data scientists and engineers are becoming the backbone of any AI-powered organization. Their skills in collecting, preparing, and managing high-quality data are indispensable. Additionally, the work in data vectorization is transforming how we interact with unstructured data. By converting PDFs, images, audio, or video into embeddings, we can ask more nuanced questions and find relevant information faster. Vector databases, while still evolving, will play a central role in this new paradigm.
The Evolution of Data Pipelines
So how are data workflows and pipelines changing in response to these new realities? Here are some of the key trends we’re seeing:
1. New Data Types & Modalities: Unstructured data like text, images, audio, and video are gaining prominence. New modalities are emerging, powered by techniques like embeddings and vector search.
2. Automation & Augmentation: Data prep and analysis are becoming increasingly automated, with tools like co-pilot assistants and auto-generated code. This is freeing up data scientists to focus on higher-value tasks. An example of a company in this domain is Keebo, which automatically optimizes Snowflake parameters and queries to save engineers time and money. Another, Typedef, backed by Pear, is working to monitor DAGs within a multi-step datapipeline to develop auto-tuning and optimizations that enhance the efficiency of pipeline execution.
3. Scalable Data Infrastructure: Data infrastructure is becoming faster and more efficient to handle the demands of AI. Vector databases are enabling fast retrieval and inference on massive datasets. “RAG-as-a-Service” is emerging to connect an organization’s proprietary data with large language models. For example, EdgeDB is an open source database that enhances PostgreSQL with hierarchical queries that are more efficient in handling AI applications with tree-like structures.
4. Collaborative Data Science: “Notebooks 2.0” — in addition to advanced platforms such as Jupyter Notebook, Databricks, and Tableau — are enabling more collaborative features and tools for accessible data science. Techniques like text-to-SQL and semantic analytics are democratizing data exploration.
5. Data Quality & Labeling: With the primacy of data quality, companies are investing heavily in data labeling and annotation. A whole ecosystem of services is emerging to provide high-quality, human-in-the-loop data labeling at scale. Synthetic data generation using AI is also being used to augment datasets. An notable example is Osmos, backed by Pear, automatically catching errors in data and removing the need for manual data cleanup.
6. Feature Stores & ML Ops: Dedicated feature stores are becoming critical to serve up-to-date features across AI models. ML Ops platforms are being adopted to manage the full lifecycle from data prep to model deployment. Versioning, metadata management, and reproducibility are key focus areas. Examples from the many existing ML Ops platforms include Vertex AI, DataRobot, and Valohai.
7. Real-time & Streaming Data: As AI is applied to real-time use cases like fraud detection and recommendations, streaming data pipelines using tools like Kafka and Flink are gaining adoption. Online machine learning is enabling models to continuously learn from new data. A prominent example is Aklivity, a company that makes a business’s real-time data streams connected to Kafka available through APIs.
8. Governance & Privacy: With AI models becoming more complex and opaque, there is a heightened focus on responsible AI governance. Tools for data lineage, bias detection, and explainable AI are being developed. Techniques like federated learning and encrypted computation are enabling privacy-preserving AI.
The Opportunity Ahead
The rapid evolution of data handling in the AI era presents a massive opportunity for data and analytics companies. Organizations will need expert guidance and cutting-edge tools to harness the full power of their data assets for AI.
From automated data prep and quality assurance, to scalable vector databases and real-time feature stores, to secure collaboration and governance frameworks — there are opportunities at every layer of the modern AI data stack. By combining deep domain expertise with AI-native architectures, data companies can position themselves for outsized impact and growth in the years ahead.
As AI continues to advance and permeate every industry, the companies that can enable high-quality, responsible, and scalable data pipelines will be the picks and shovels of this new gold rush. The future belongs to those who can tame the data beast and unleash the full potential of AI. Are you building in this space? Let’s talk.
Acknowledgements
I’d like to thank Avika Patel and Libby Meshorer for their contributions to this post. Visit our AI page to read more about the 16 spaces we’re excited about.