We recently launched a dedicated AI track to the PearX program and have received a great response. Founders often ask us for guidance on how to build a moat for their AI startup. There are many aspects to this question but to kick things off, we are sharing a presentation I gave at SF Tech Week that covers background on the emergence of Generative AI, the highest priority areas of application particularly in enterprises, and what we believe enables a ‘moat’ for AI startups.
Generative AI Tech Stack Presentation at SF Tech Week
Generative AI is a game changing technology for humanity. A quote from one of my heroes, Professor Fei Fei Li at Stanford, and also was head of AI at Google Cloud for a while captures the excitement well:
“Endowing machines with generative capabilities, has been a dream for many generations of AI scientists”
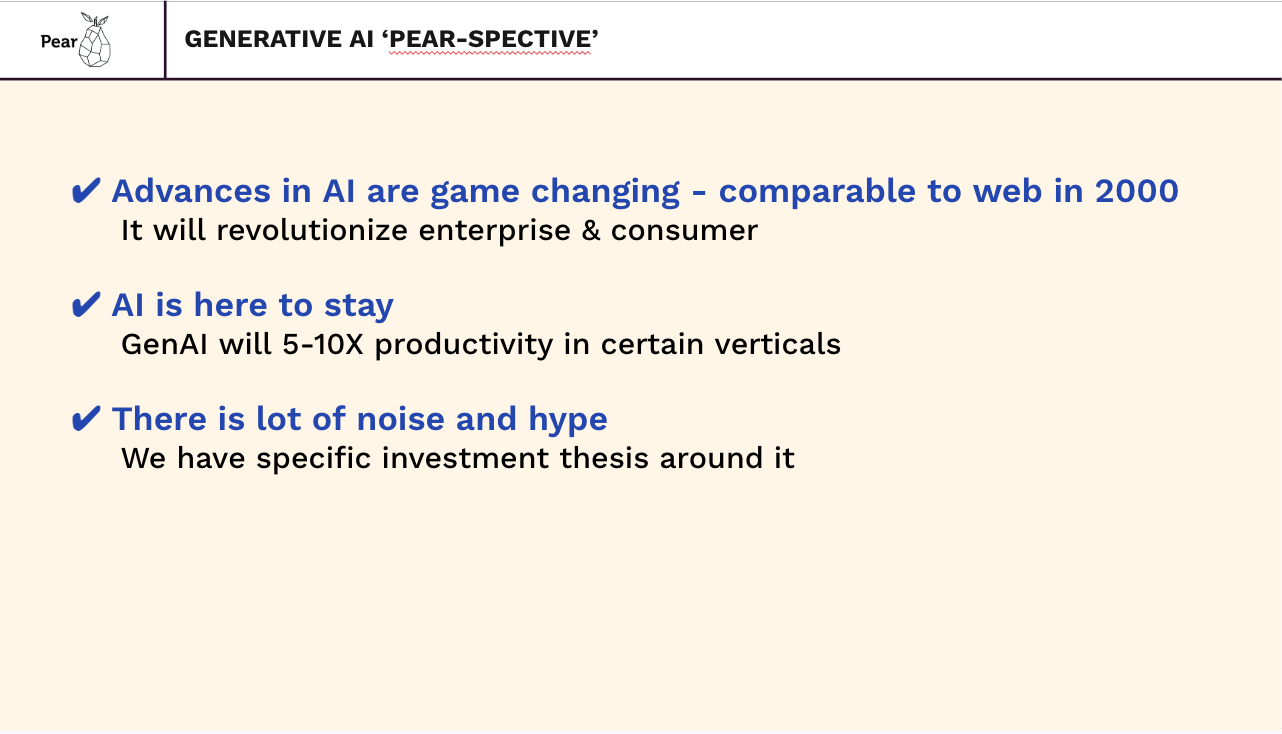
Seminal technologies which have led to the recent Generative AI breakthroughs include Cloud computing and within that advancements in GPUs, Kubernetes and open source frameworks like PyTorch provide an efficient and widely accessible substrate for model training and inference.
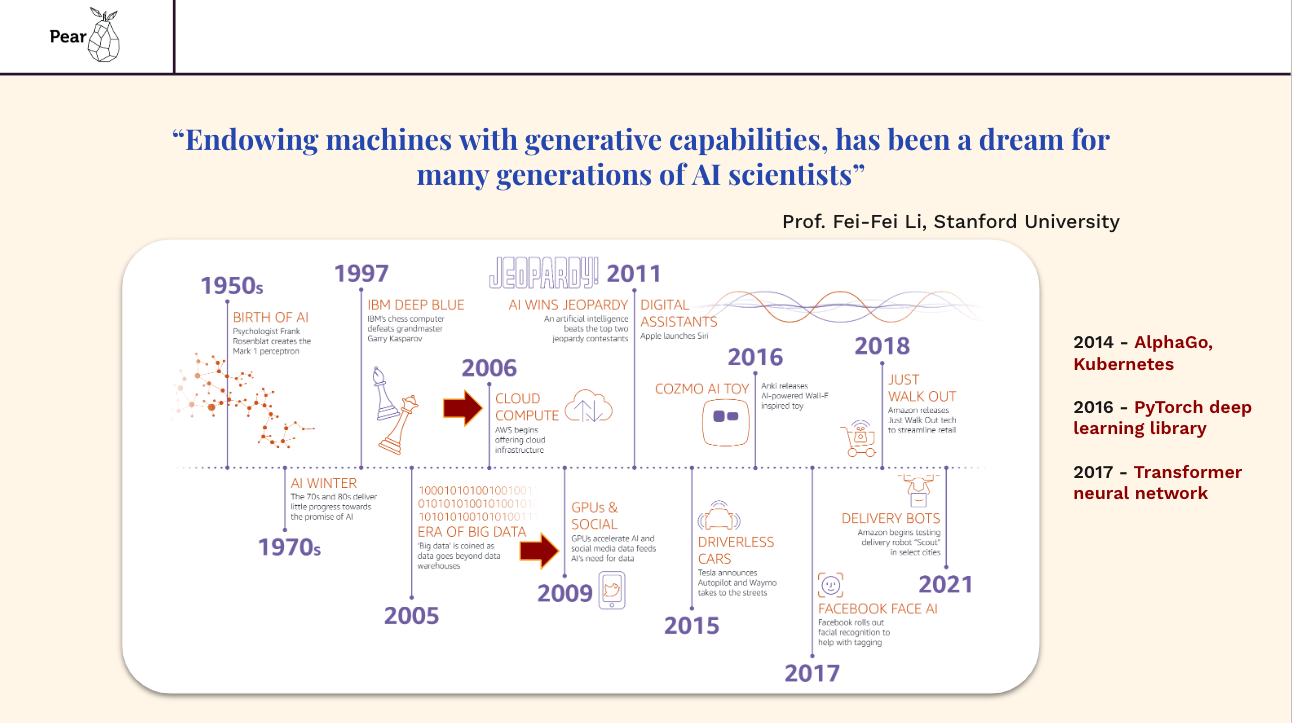
Research breakthroughs on the transformer neural network, its use on internet scale datasets and recent advancements in AI alignment are at the heart of most of the Generative AI capabilities today.
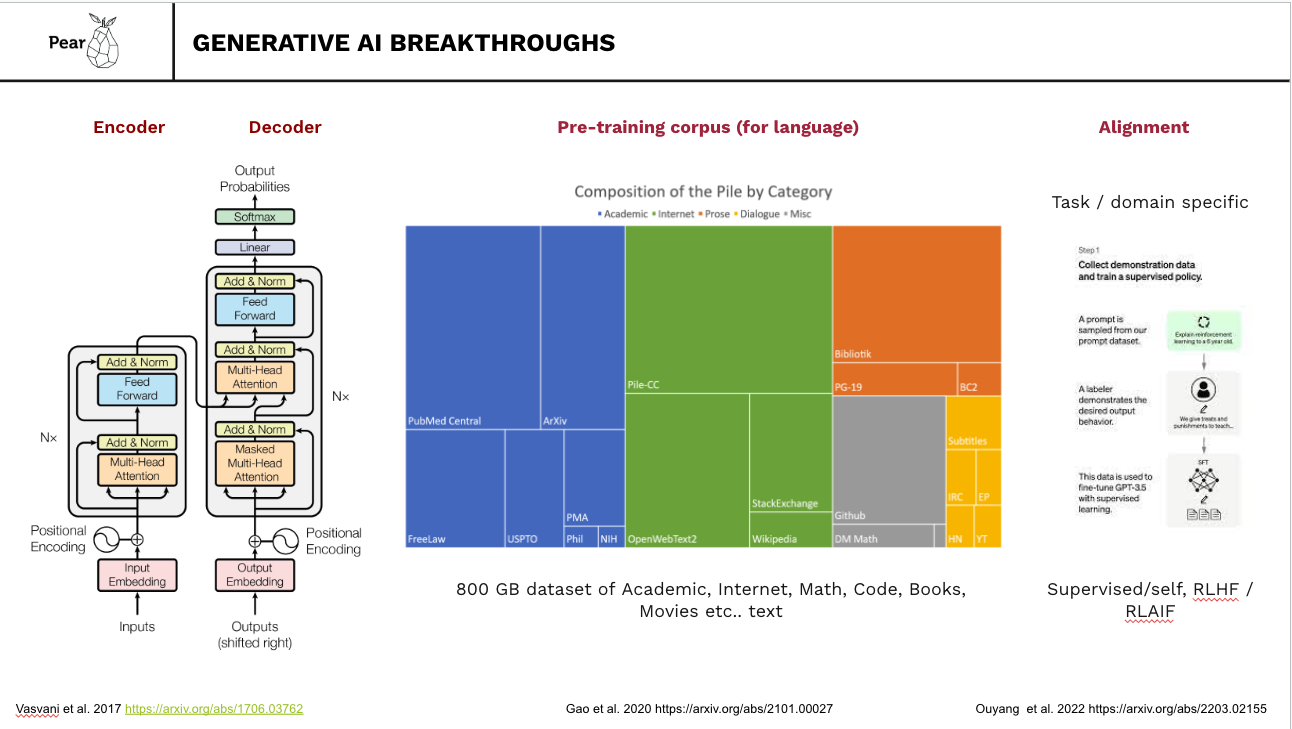
By no means are we at a peak yet, as research continues to improve efficiency at the hardware, software and services layers. Most interestingly to increase context lengths and optimize AI application architectures for accuracy, latency, and reliability. We cover some of these topics in depth in our Perspectives on AI fireside series.
It is clear that Generative AI techniques apply to multiple modalities. There has been a steady stream of models, both open source and proprietary in the major areas of NLP, Image, Video, Voice and also physical synthesis of Proteins.
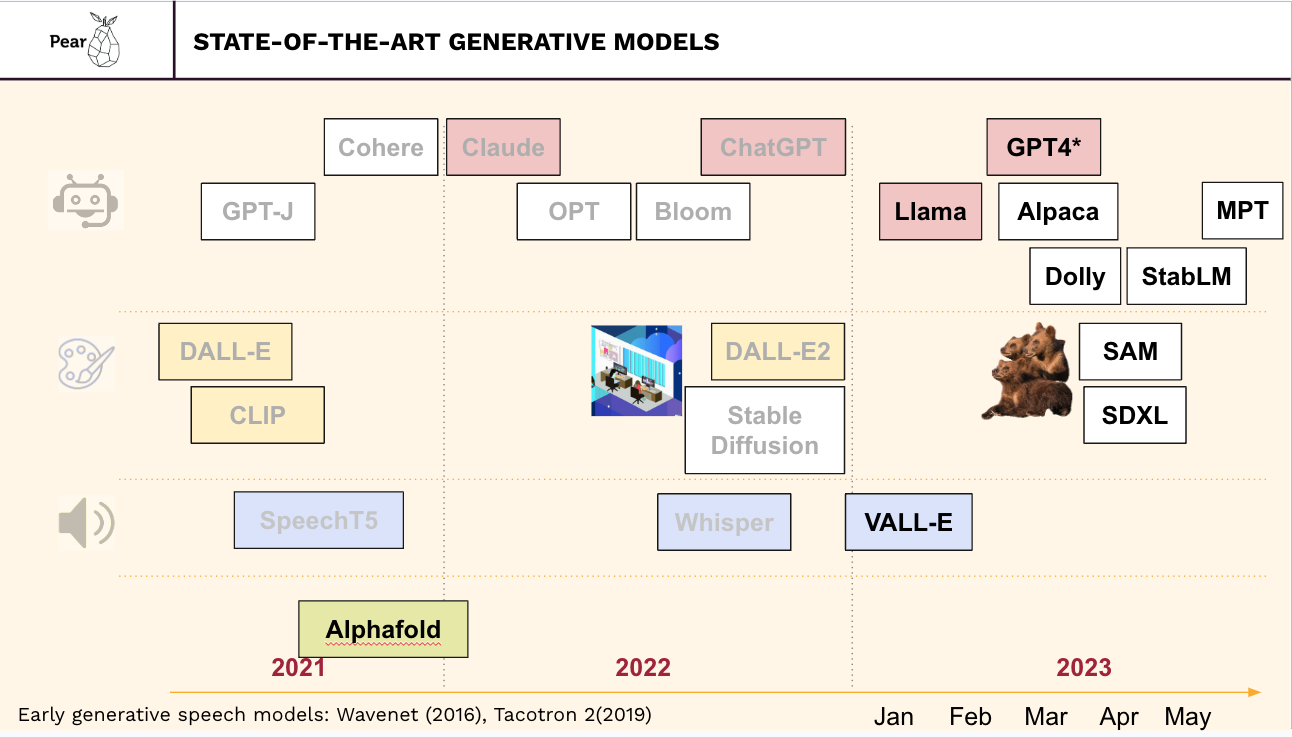
Applications to both consumer and enterprise software abound and are already starting to change the shape of what software can do. We highlight some of the opportunities to build vertical and horizontal applications as well as tooling and infrastructure.
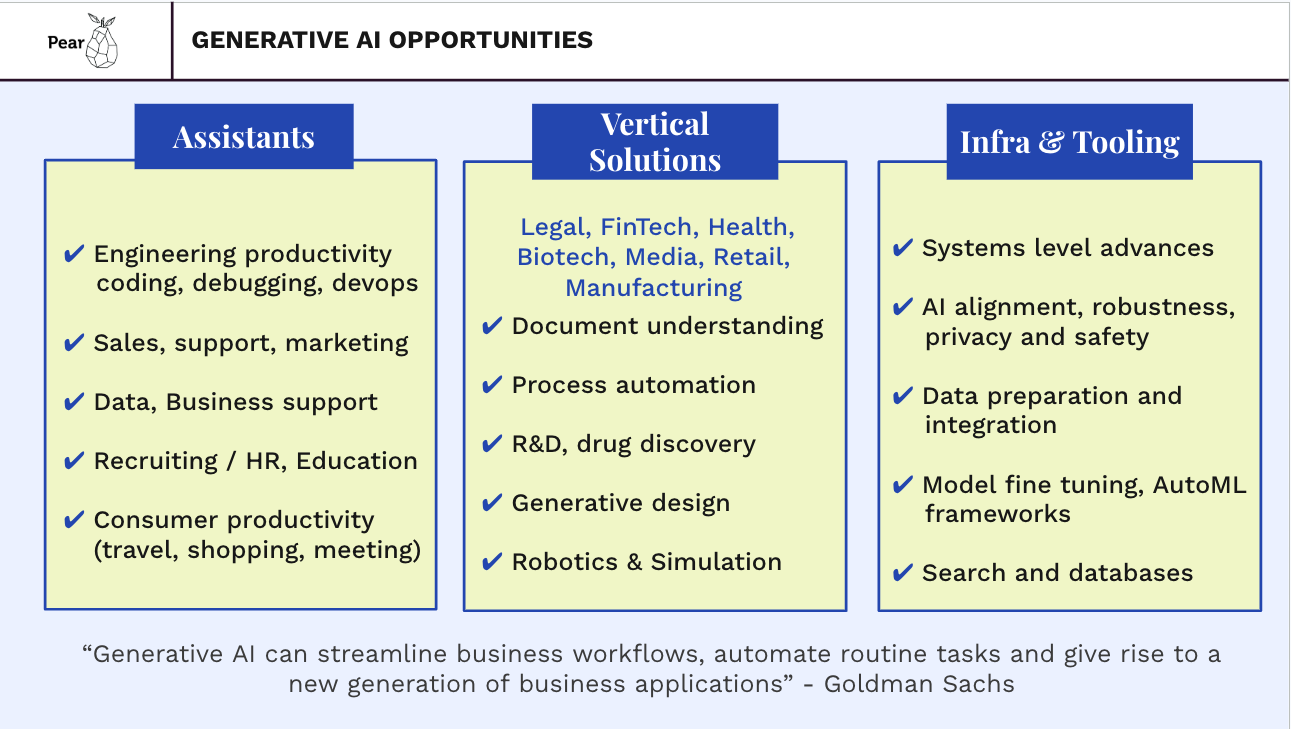
Of course there is hype when it comes to Generative AI, and in some sense it is almost too easy to create new functionality by building a thin layer over a foundation model that somebody else has built. While there are some businesses to be built in that way, for a venture scale business, we posit that a deeper moat is required to build. A large business that benefits rather than crumbles from rapid evolution of technology at the lower layers of the AI stack requires several moats.
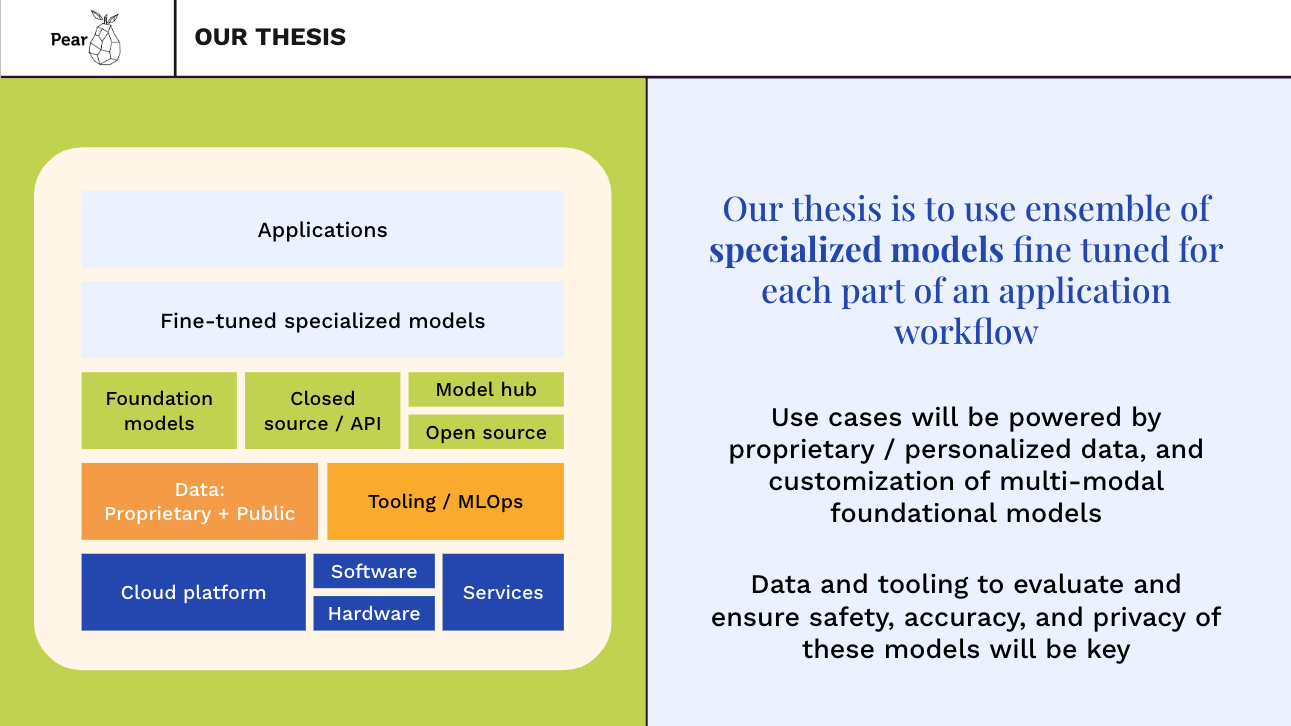
Our thesis is that Applications will be composed of ensembles of specialized models, not just foundation models, but specialized models that are customized via fine tuning or in-context learning or a range of other techniques to complete part of a use case or workflow. These specialized models should utilize proprietary data specific to a domain and help to personalize the output of the application as well as ensure accuracy. A by product may also be lower cost to serve. Overall such an architecture will be a way to build lasting value and be more immune to disruption.
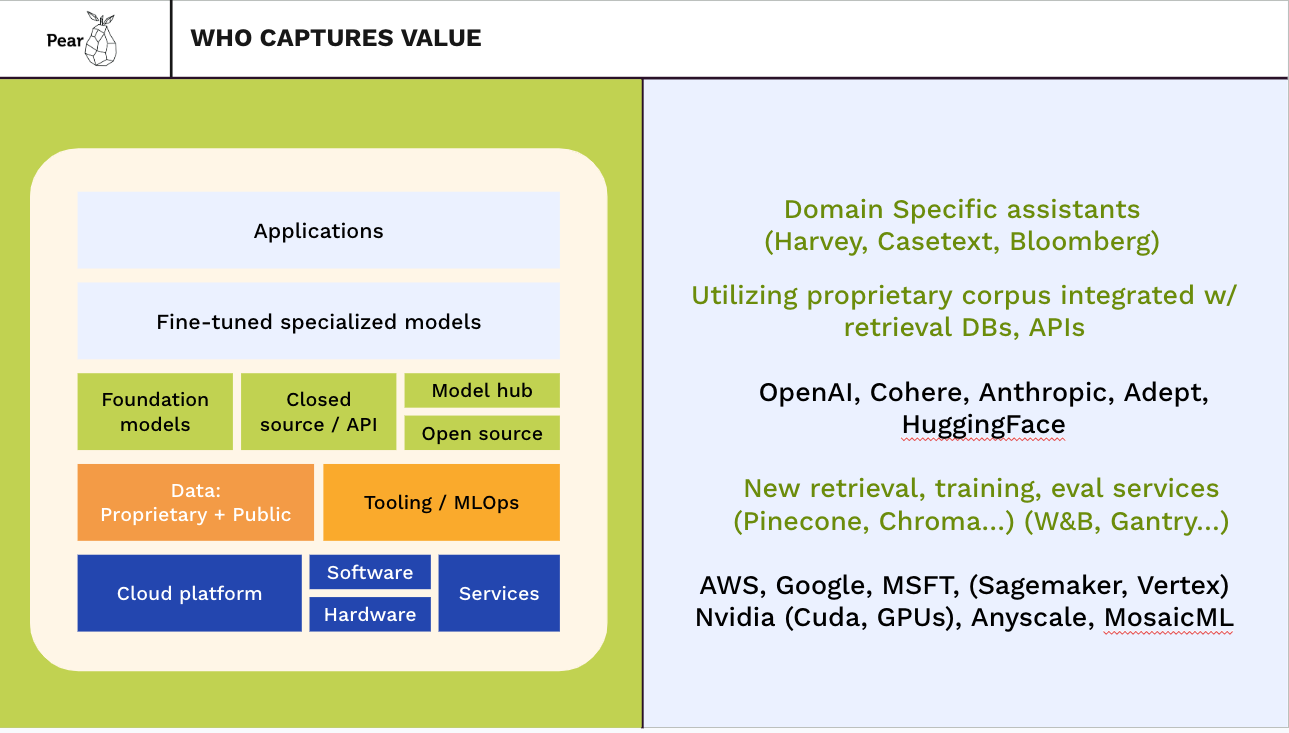
Tooling and infrastructure supporting the development of new applications of this kind is second part of our investment thesis. In particular, data and tooling companies to evaluate and ensure safety, accuracy, and privacy of these applications will be in demand. Lastly a few new infrastructure companies and capabilities will advance the development of these applications. We see emerging companies at every layer of the AI stack (slide 9). With that thesis in mind, building a moat is fundamentally not that different in AI than in any other emerging space (slide 10).
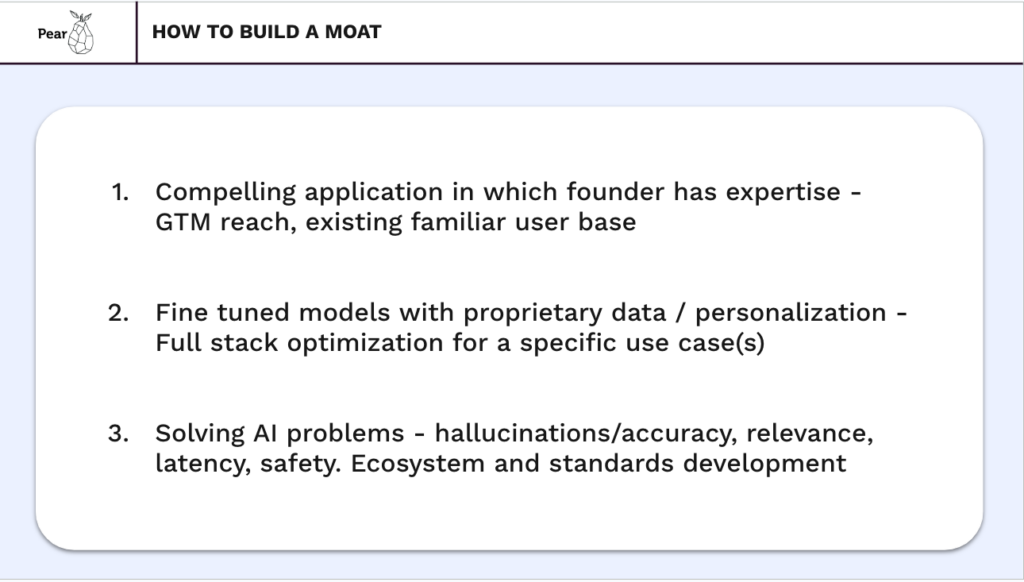
Enterprise readiness for adoption of AI is arguably higher than it has ever been with the widespread acceptance of cloud computing, API integrations, and existing investments in data analytics teams and software. The hurdles to enterprise adoption are also not new, these are the same requirements that any cloud service has to meet, with perhaps a stronger need for ease of use and simplicity given the lack of existing AI/ML talent.
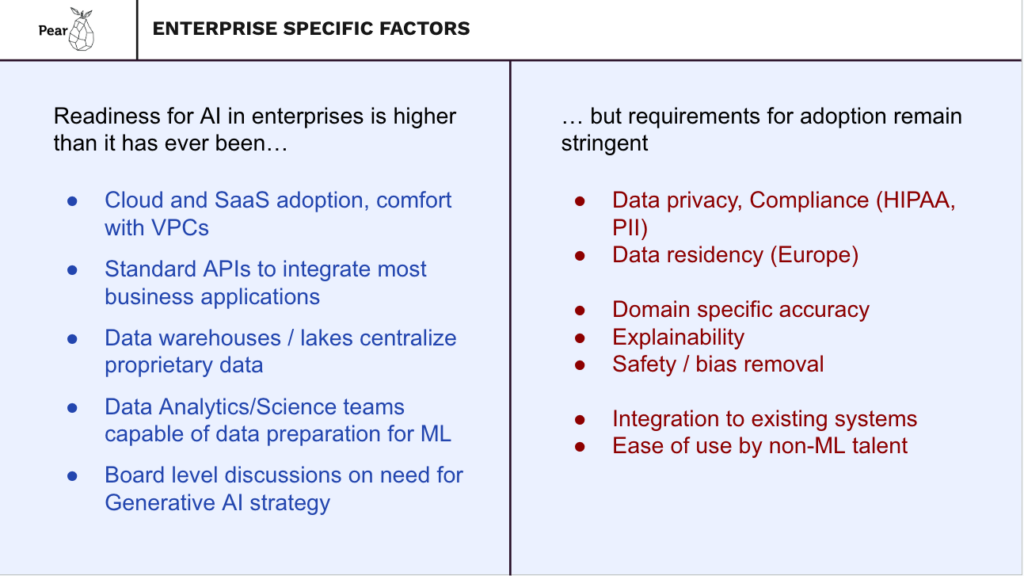
We conclude by quoting what many others have already said, that this is a great time to start a company!